Cos4Cloud is a Horizon 2020 project that uses deep Machine Learning and video recognition to design citizen science technologies.
About
Cos4Cloud (Co-designed citizen observatories for the EOS-Cloud) is a European Horizon 2020 project aiming to boost citizen science technologies by designing and implementing services. The project will design and prototype these new services using deep Machine Learning (ML), automatic video recognition, and other cutting-edge technologies. The project, started in November 2019, is coordinated by the Instituto de Ciencias del Mar (ICM)-CSIC from Spain. The consortium is composed of 16 partners from 8 countries.
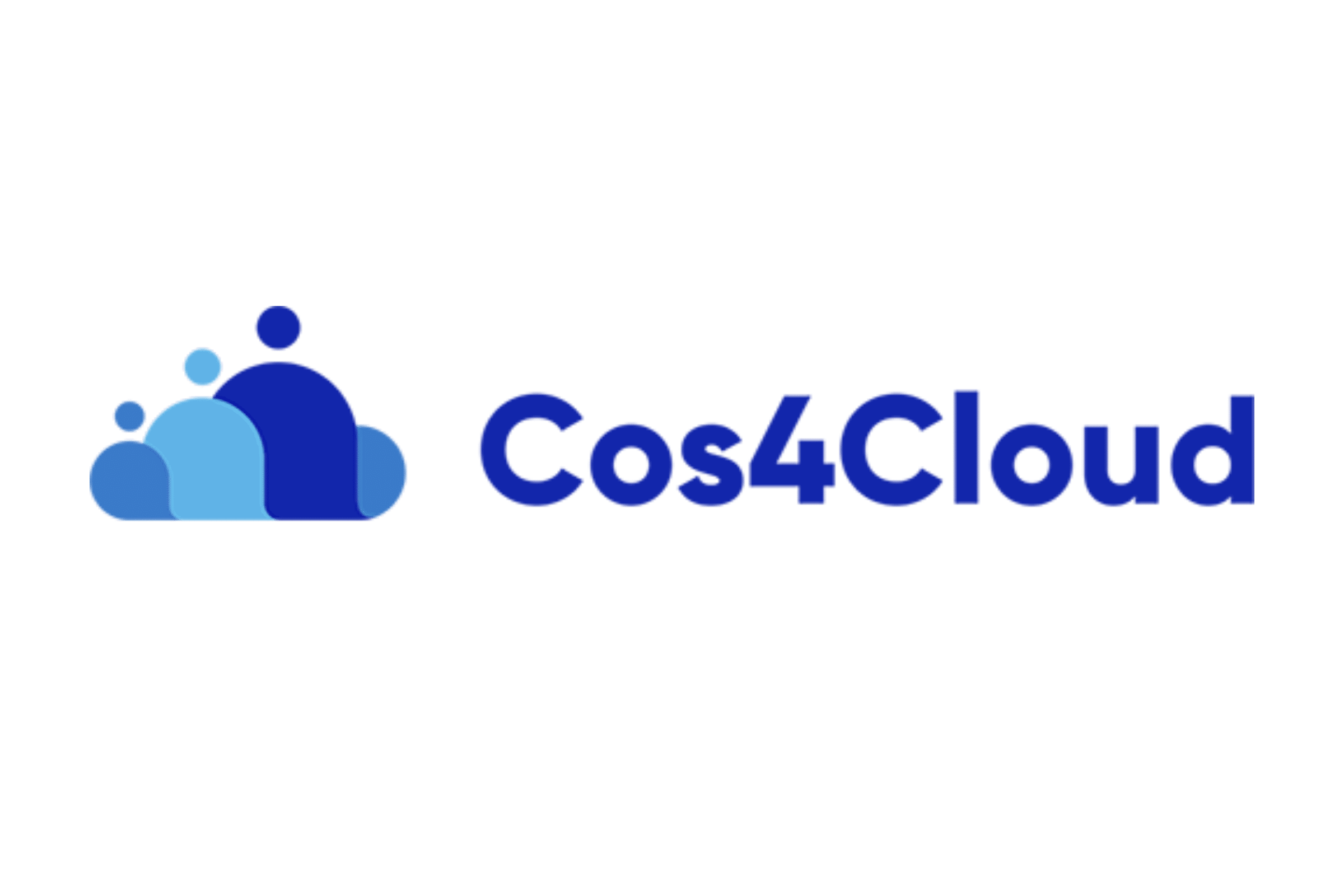
The Challenge
One of the biggest challenges of citizen science is the quantity and quality of data, as well as maintaining the citizen observatories used to collect this data. Cos4Cloud addresses these challenges by developing 13 technological services to improve citizen science platforms. These services will be co-designed with key stakeholders and carefully tested with end-users, and available in EOSC.
Specifically, the Cos4Cloud project aims to create a federation of Citizen Science portals and an expert Portal that exchanges citizens’ observations for quality assurance. The scientific objective is to study whether the developed extension to the OGC Sensor Things API for Citizen Science is fit for the purpose of large data sets coming from different domains such as environmental and biodiversity.
MOBIS
To support this challenge, EGI contributed by offering federated and distributed resources to host and deploy the back-end of the MOBIS (Mobile Observation Integration Service) service. From a technical point of view, MOBIS collects and processes data from different mobile observatories (most of them smartphones with or without sensors). For this reason, it can be used as a back-end service for (citizen-) science apps with a single login. The service offers a very intuitive, user-friendly interface that allows users to start collecting valuable data using the smartphone sensors and images (e.g. air pollution, see aerosols in the sky, and produce a picture of lichens using the same app). The MOBIS service is provided by DDQ: Pocket Science, a small Dutch company specialised in the development of mobile-based (citizen-) science applications with research partners worldwide. The company also offers smartphone back-ends to facilitate mobile cloud computing, machine learning, user notifications, storage and offline synchronisation for working in remote areas.
The Solution
Cos4Cloud uses:
- the EGI Cloud Compute and the cloud-based EGI Online Storage to deploy the back-end of MOBIS.
- the EGI Check-In to enable users’ registration and authentication mechanisms.
- technical consultancy to profit from EGI solutions.
Involved Providers
- A Service Level Agreement (SLA) with Cos4Cloud was agreed with EGI in July 2021.
- The Institute of Physics of Cantabria (IFCA), a Joint Centre with the combined effort of two institutions, Spanish National Research Council (CSIC) and University of Cantabria (UC), represented by CSIC in the EGI Council, has allocated 300 vCPU cores, 100 GB of RAM and 1TB of storage.
Impact
The impact of MOBIS is to facilitate the interest and participation of multiple users in science, allowing them to collect and combine all sorts of useful information from photographs or from low-cost sensors linked to a mobile website or a native app platform. In the long run, EGI and DDQ: Pocket Science are planning to establish a business partnership to sustain the further development of the service by sharing a completely open set of cross-disciplinary data that can be sustained and grow over time.
registered in the cos4cloud-eosc.eu VO
consumed over the last two years