EMPHASIS is the infrastructure for plant phenotyping that enables researchers to use facilities, resources and services for plant phenotyping across Europe.
About
The objective of EMPHASIS is to go towards the implementation of an e-infrastructure for plant phenotyping. The beneficiaries of this e-infrastructure are the European plant phenotyping community and partners of the EPPN2020 network and the EMPHASIS (European Infrastructure for Multi-scale Plant Phenomics and Simulation) infrastructure. The main partners involved are: INRAE, the University of Copenhagen (UCPH), the University of Helsinki (UHEL), and the Wageningen University & Research and Utrecht University.
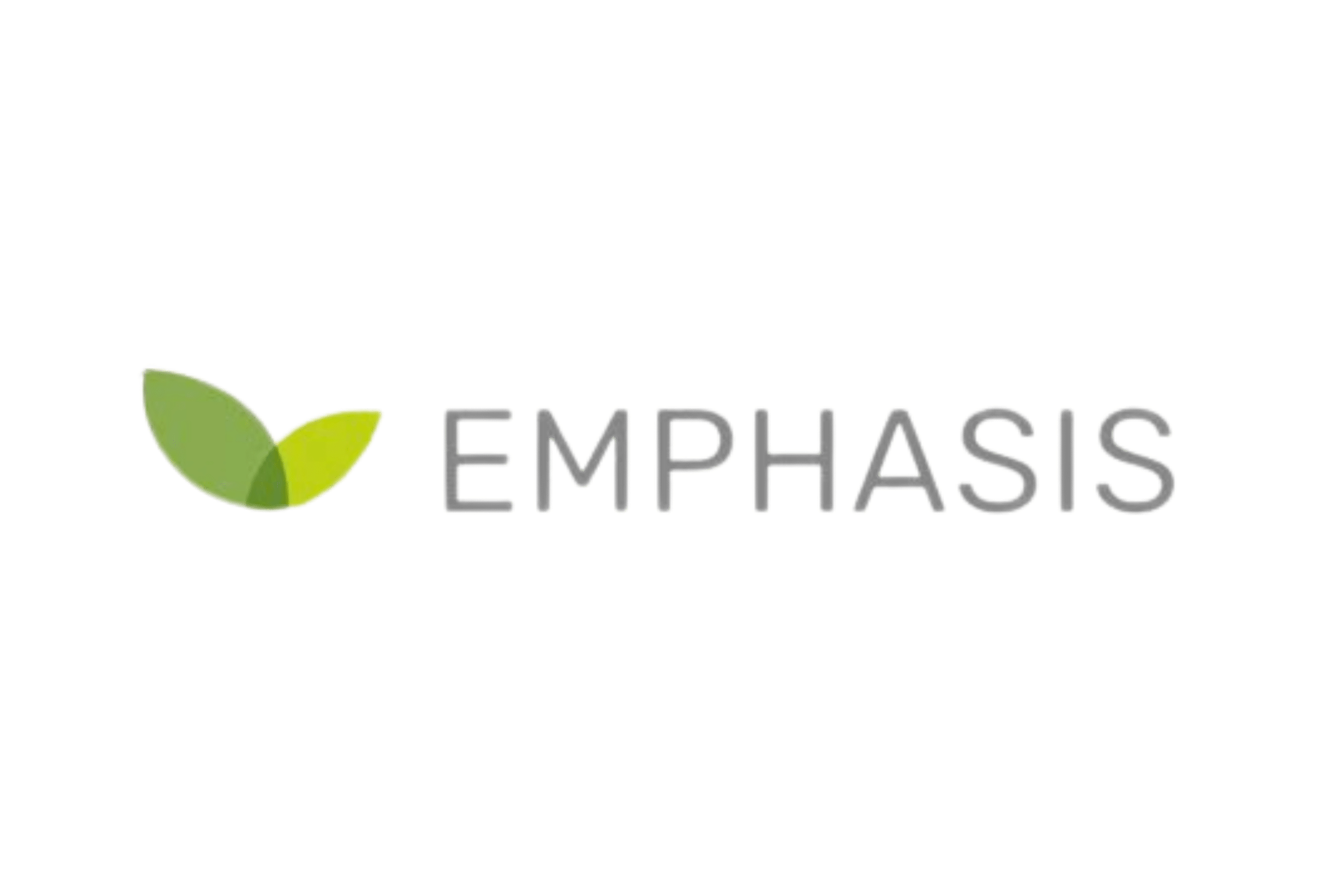
The Challenge
In recent years, technological progress has been made in plant phenomics (major improvements concerning imaging and sensor technologies). High-throughput plant phenotyping platforms now produce massive datasets involving millions of plant images concerning hundreds of different genotypes at different phenological stages in both field and controlled environments. Networks of sensors also measure environmental conditions in real time. The ongoing robotization of experimental processes foreshadows an explosion in the volume and complexity of the data produced by the different research facilities.
Various initiatives have helped to structure the European phenotyping landscape (EMPHASIS, EPPN) and enable researchers to use facilities, resources and services for plant phenotyping across Europe. However, among these services, the data services needed to be improved. Thus there was a need to build a federated and interoperable e-infrastructure allowing researchers to share and analyse phenotyping data.
The Scientific objectives were:
- analyse raw data (thousand of images) and extract variables of agronomic interest;
- combine data coming from several sources including large genetic and environmental variabilities and run meta analysis in order to predict the response of genotypes and species to current and future climate scenarios;
- structure the data (raw and processed data) in a way that it can be shared and reanalyzed by a wide scientific community: using a standardised, unique and unambiguous identification of objects involved in experiments, enriching datasets with knowledge and metadata enabling the reuse of data and meta-analyses;
- evaluate online deep learning and iterate with shared annotation and training/testing.
The Computing objectives were:
- Data management:
- Data integration between infrastructures/platforms (and other systems);
- Data management and integration of heterogeneous multi-dimensional data from multiple sources;
- Data export to computing and modelling platforms;
- Dataset compatibility and interoperability between infrastructures;
- Production pipeline for creation of shared, and FAIR-compliant datasets
- Connection over BrAPI, https://brapi.org/
- Data analysis:
- access to a robust infrastructure for storage and data processing of large datasets;
- Integration of innovative computing services provided by the EOSC portal (including deep learning);
- Use cases of computer vision including all main informational tasks such as classification, object recognition, segmentation and regression.
- Data (or services) publishing:
- Increase visibility of our services by publishing them into the EOSC portal.
- for our published datasets, we are looking for a long-term dataset repository like dataverse.
The implementation of the data management part of this infrastructure is based on a storage layer based on the open-source Phenotyping Hybrid Information System PHIS (Neveu et al, 2019; www.phis.inrae.fr). PHIS is an ontology-driven Information System designed for Plant Phenomics, that allows to enrich datasets with knowledge and metadata enabling the reuse of data and meta-analyses. It interoperates and integrates data into external resources (e.g. modelling platforms or external databases) and provides FAIR data. PHIS is designed to store, organise and manage highly heterogeneous (e.g. images, spectra, growth curves) and multi-spatial and temporal scale data (leaf to canopy level) originating from multiple sources (field, greenhouse). PHIS has already been deployed in several French platforms for high throughput plant phenotyping including field and controlled conditions within the PHENOME-EMPHASIS project.
The Solution
A storage layer based on the open-source Phenotyping Hybrid Information System PHIS is proposed to store, organise raw data and capture data provenance. The EGI Cloud Compute is used to host three PHIS instances.
The EGI Check-In has been integrated as the default authentication system. The connection to PHIS instances is thus proposed with this authentication system in addition to the usual connection.
The online storage service provided by FranceGrilles FG-iRODS is used. EGI DataHub solution will be tested when we will encounter a use case interested in.
EMPHASIS uses the Deep Hybrid DataCloud portal to host DL models developed by Angers University.
DEEPAAS-API is a web service containing modules with a Deep Learning model. In each module, the model can be used for two tasks. A user can train the model with his own data. A user can make an inference with his data from the model. In April 2023, the web service contained 24 modules such as Dogs breed detector, Body pose detection, or Plant classifier. The GPU resource is very useful for the computation time of a Deep Learning model design. DEEPAAS-API offers a GPU for free. The combination of several GPUs is in progress.
During the EGI-ACE project, EMPHASIS has extended the number of models adapted to plants with Apple tree blossom image segmentation and apple detection. More generically, EMPHASIS has developed the possibility to improve a model. A user can now manually improve the annotation of masks given by inferring a model with the napari-pixel-correction plugin of the napari software. These improved masks can be stored in a directory. At the moment, this directory is located on Google Drive. When the number of data in this directory is large enough, it will be possible to re-train the model by reading the training curves and metrics values.
EGI notebook: training material on how to use the tool developed during the EGI-ACE project were provided.
A web page exploiting JupyterLab for writing programming code in notebook paper. It is possible to write scripts in R, MATLAB and Python language. EGI notebook gives you a session where the default session contains 2 CPU cores, 6 GB RAM and 20 GB of personal storage space. From its own session, user can run data analysis or data processing. Its data is stored into his own personal storage. In particular, EGI notebook gives the possibility to exploit a GPU resource if heavy data processing or data analysis may be run. As part of the Phenomics data platforms workshop, participants were granted access to the EGI-notebook. This enabled them to practise image and data processing using both Python and R languages.
The workshop “Phenomics data platforms” aimed to promote FAIR data management practices and PHIS adoption among image-data users. It also covered data processing tools and methods in EGI platforms.
It consisted in 2 days of online Workshop + one day hands-on event on how to use JMP and/or R for image-based data processing and on PHIS.
During the workshop, a dozen lectures and showcases were given.
More than 40 participants from the NAPPI and NordPlant networks joined it.
Services Provided by EGI
Impact
hosted by the EGI Cloud
on Phenomics data platforms
from the NAPPI and NordPlant networks
available on the DeepHybrid datacloud
limited at one for any model deployed
205 in use
700 GB in use